Ready, set... go š
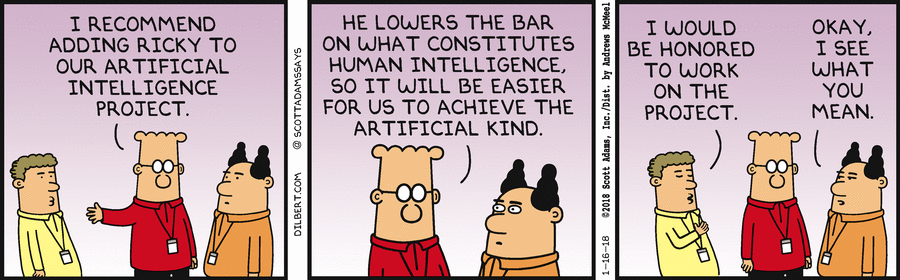
Woohoo, you made it ā thanks so much for reading the inaugural Digital Coffee āļø. I took a bit of a break from regularly posting content online for the past year or so. This newsletter is an attempt to get back into the flow.
If you do have a minute please, Iād be grateful for any feedback. This is all an experiment; that said, I am confident that youāll takeaway something of use each week.Ā
Now for the typical please share . I think there are ~50 people signed up. If you think of anyone who would enjoy reading, just ping them the digitalcoffee.substack.com link. Also, feel free to share on LinkedIn, Twitter etc. Thank you so much š
Now onto the content! š
This weekās espresso shots
Beyond Meat š„©Ā
In a big year for IPOs, with Uber, Lyft, Pinterest and Zoom, to name just a few, already having gone public and more companies waiting in the wings, it is ironic that it is not a tech company, but a food company, Beyond Meat ($BYND), that is performing best. The stock has grown seven-fold since launch. Aswath Damodaran has a great analysis of the business here.
Mental Models š§ Ā
In previous newsletters that Iāve drafted I always forget to mention my go-to websites, thinking that everyone has discovered them already. To buck this trend, I want to first recommend a site that Iāve visited weekly for quite some time, Farnam Street. Shane (the author) has a great podcast, he also summarises mental models well. As for which mental model to focus on first, Iād encourage anyone who wants to be more creative to read āthinking from first principlesā.Ā
Currently reading š
āInfluenceā by Cialdini; In many situations, we humans like to avoid thinking about how we should react by using predictable shortcuts to guide our decisions. Professionals like advertisers, con artists and salespeople take advantage of these preprogrammed human reactions to elicit the response thatās in their best interests, not ours. Specifically, they leverage the principles of reciprocation, scarcity, consistency, social proof, liking and authority. Since we cannot stop using these shortcuts that mostly serve us well, we must instead learn to defend ourselves against the manipulators who abuse them.Ā
Making money š°Ā
Many people think you have to build the next Facebook in order to get wealthy online. Over the past five years or so Iāve been focusing on micro-businesses. There are millions of websites and digital businesses generating tens of thousands of dollars in revenue each month. Instead of trying to think of the unicorn, starting thinking about how to nurture a good āstable of horsesā. A diversified portfolio. And the good news is, by taking this approach, youāll most likely not have to take outside capital ā meaning whatever cash is generated is yours to keep, or reinvest! One website (and podcast) that I couldnāt do without is Empire Flippers.Ā
Coffee & chats
Start building š©āš§
After spending just over a year building artificial intelligence products, I wanted to share some thoughts...
Regardless of whether youāre in the tech space or not, I would recommend you build a simple AI application. Itās really not as daunting as it sounds.
Why? Every single industry is about to be (or rather is currently being) impacted by artificial intelligence ā think of the impact in an order of magnitude similar to when the internet first launched. Meaning your job, your company, the products you buy, how you conduct your normal day-to-day life, will all be impacted by the prevalence of AI.Ā
When I say build your first AI application, I actually mean build a machine learning application. What is machine learning? And how does it differ from artificial intelligence? Ā
Machine learning is a subset of AI. That is, all machine learning counts as AI, but not all AI counts as machine learning. For example, symbolic logic ā rules engines, expert systems and knowledge graphs ā could all be described as AI, and none of them are machine learning. One aspect that separates machine learning from the knowledge graphs and expert systems is its ability to modify itself when exposed to more data; i.e. machine learning is dynamic and does not require human intervention to make certain changes. That makes it less brittle, and less reliant on human experts.
The ālearningā part of machine learning means that ML algorithms attempt to optimise along a certain dimension; i.e. they usually try to minimise error or maximise the likelihood of their predictions being true. This has three names: an error function, a loss function, or an objective function, because the algorithm has an objective⦠When someone says they are working with a machine-learning algorithm, you can get to the gist of its value by asking: Whatās the objective function?
How does one minimise error? Well, one way is to build a framework that multiplies inputs in order to make guesses as to the inputsā nature. Different outputs/guesses are the product of the inputs and the algorithm. Usually, the initial guesses are quite wrong, and if you are lucky enough to have ground-truth labels pertaining to the input, you can measure how wrong your guesses are by contrasting them with the truth, and then use that error to modify your algorithm. Thatās what neural networks do. They keep on measuring the error and modifying their parameters until they canāt achieve any less error.
They are, in short, an optimisation algorithm. If you tune them right, they minimize their error by guessing and guessing and guessing again.
Building a machine learning application can be broken down into the following steps:Ā
1. Understand and define the problem
2. Analyse and prepare the data
3. Apply the algorithms
4. Reduce the errors
5. Predict the result
Rather than go through all of these in this email; Iāll leave it to the experts... Iāve completed quite a few online courses, and in my opinion, the one that is most ābeginner friendlyā would be Machine Learning Mastery.
Some interesting links
Upgrade your memory with a surgically implanted chip
Anxiety, an interactive storyĀ
Thatās it folks, I hope you enjoyed this first newsletter. Again, if you did ā please share it with your friends! See you next Sunday šĀ